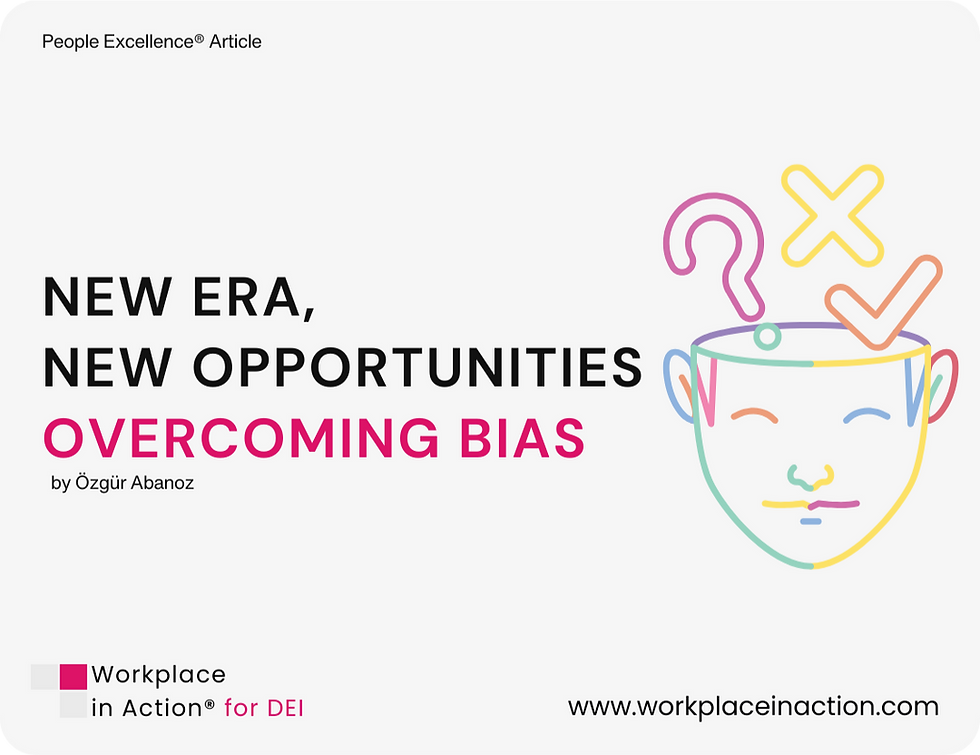
People Excellence® Magazine Issue 02-2024
In an era where data-driven decisions are pivotal, data bias poses a significant challenge, particularly in professional environments. Data bias undermines the integrity of decision-making processes, perpetuates inequalities, and hinders the realization of Diversity, Equity, and Inclusion (DEI) goals. We must try to understand the nature of data bias, its impact on professional fields, and strategies to mitigate its effects to foster a more inclusive and equitable workplace.
Data bias occurs when the data used in decision-making processes is skewed, leading to outcomes that favour certain groups over others. This can happen due to various reasons, such as historical bias, sampling bias, and measurement bias. Historical bias, for instance, is evident when data reflecting past discriminatory practices influences current decisions. Sampling bias occurs when the data collected does not represent the entire population. Measurement bias happens when there are errors in how data is collected, recorded, or interpreted. An illustrative example of historical bias is found in recruitment algorithms. If an algorithm is trained on historical hiring data that predominantly reflects male candidates, it may inherently favour male applicants, perpetuating gender bias in hiring practices.
Data bias can have profound implications in many professional fields, where decisions often rely heavily on data analytics and automated systems. Biased algorithms can lead to discriminatory hiring practices, excluding qualified candidates from underrepresented groups. For instance, a company might use an AI-driven recruitment tool that inadvertently filters out resumes from women or minorities due to biases in the training data.
Data-driven performance metrics may reflect inherent biases, disadvantaging certain employees and affecting their career progression. For example, performance evaluation systems that rely on biased data might systematically undervalue employees' contributions from minority groups.
Credit scoring models and loan approval processes in financial services can inadvertently favour specific demographics, perpetuating economic disparities. For example, a credit scoring algorithm based on historical data reflecting discriminatory lending practices may unfairly penalize minority applicants. Similarly, bias in medical data can result in unequal treatment recommendations, exacerbating health inequities. An example of this is found in healthcare algorithms that are less likely to recommend advanced treatments for minority patients based on biased data.
The consequences of data bias in these contexts are ethical and practical, as organizations risk losing diverse talent, facing legal challenges, and damaging their reputations. Addressing data bias requires a multifaceted approach that combines technological, procedural, and cultural interventions.
One effective strategy is ensuring diverse data collection. This involves collecting data from diverse sources and continuously updating datasets to reflect current realities. For example, a company could ensure that its recruitment data includes a balanced representation of gender, race, and other demographics. Regular bias audits of algorithms and data-driven systems can help detect and correct biases in predictive models. Inclusive design teams with diverse perspectives can identify potential biases that homogenous teams might overlook.
Transparency and accountability are also crucial. Maintaining transparency in how data is collected, processed, and used, as well as implementing accountability measures, ensures that biases are addressed promptly and effectively. For instance, organizations could publish regular reports on their data practices and the steps to mitigate bias. Other essential steps include providing bias training for employees on recognizing and mitigating data bias and establishing ethical guidelines and policies for data usage that prioritize fairness and inclusivity. This could consist of training sessions on unconscious bias and the ethical use of data analytics.
Some notable case studies highlight successes and challenges in addressing data bias. For instance, a leading tech company identified gender bias in its recruitment algorithm, favouring male candidates. By conducting a comprehensive audit, the company re-engineered the algorithm to eliminate gender-based disparities, resulting in a more diverse workforce. Another example is a healthcare provider that discovered racial bias in its treatment recommendation system, which was less likely to recommend advanced treatments for minority patients. By integrating diverse datasets and employing fairness-aware algorithms, the provider improved the equity of its treatment recommendations.
Despite these successes, challenges remain. Bias detection and correction can be technically complex, and there often needs to be more resistance to change within organizations. Additionally, balancing the trade-off between model accuracy and fairness requires careful consideration. For instance, ensuring an accurate and fair algorithm may involve difficult trade-offs, such as sacrificing some degree of predictive accuracy to achieve greater fairness.
Addressing data bias is a technical challenge and a moral imperative for professionals committed to DEI. By adopting comprehensive strategies to mitigate data bias, organizations can ensure fairer, more inclusive decision-making processes that align with their DEI goals.
Integrating ethical considerations into data analytics will be crucial in shaping a more equitable and just professional landscape as we move forward. Embracing these changes aligns with ethical standards and enhances organizational performance by leveraging diverse perspectives and fostering a more inclusive work environment.
The implications of data bias are particularly significant for information technology (IT) and STEM fields. In these sectors, where innovation and precision are paramount, biased data can lead to flawed technological solutions and hinder scientific advancements. For instance, biased training data can result in algorithms perpetuating inequalities in machine learning and artificial intelligence. IT professionals and STEM researchers must prioritize fairness in their data practices by implementing diverse data collection methods, conducting regular bias audits, and ensuring inclusive representation in design and development teams.
Furthermore, incorporating ethical considerations into the STEM education curriculum can help future professionals recognize and address data bias from the outset of their careers. By fostering a culture of inclusivity and ethical responsibility, the IT and STEM fields can create equitable and unbiased technological solutions that benefit all members of society.
Comments